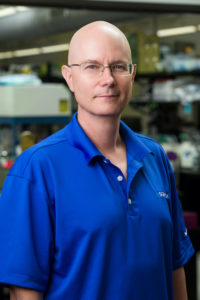
By Richard Fox, PhD
Since the first reports of CRISPR (clustered regularly interspaced short palindromic repeats)-based gene editing came out eight years ago, the enthusiasm for applying it to any number of scientific challenges has been palpable in the research community. Unfortunately, this eagerness belies the challenges of deploying CRISPR workflows.
While CRISPR methods are indeed tremendously promising, it is important that we acknowledge the serious technical limitations that exist today. Until we face these problems directly, we will not be able to realize the true potential of this remarkable scientific tool.
In laboratories dedicated to the use of CRISPR, there is sufficient expertise to deal with many of these limitations. But in the vast majority of labs, scientists may struggle to get CRISPR pipelines up and running, particularly at scale. The design process required to build guide RNAs, cassettes and nucleases can be more art than science. At best, it involves fussy, unreliable techniques. At worst, it can be the insurmountable obstacle that prevents many labs from implementing CRISPR at all.
Trackability is another hurdle. It’s essential for good science to record each edit made and then monitor those changes. But this tracking process is increasingly difficult as researchers attempt more complex edits such as multiplex (combinatorial) editing. The lack of an accurate record of all changes made is a serious shortcoming as we look to expand the use of CRISPR, particularly for human health applications.
While limitations in design techniques and trackability must be addressed, I believe that the single biggest flaw in today’s CRISPR workflows is the inability to scale. This is truly the make-or-break issue facing CRISPR right now. If we cannot overcome the scalability problem, it will be impossible to deploy this tool to deal with the most pressing challenges we face in climate change, public health and so much more.
Large-scale biology applications — such as systems biology or biological engineering — often present us with nearly limitless possibilities to test to find improved solutions. Despite the advances made in synthetic biology, we simply don’t know enough about Nature’s rules to engineer biology from first principles the way you would design a circuit or an airplane. Because so much is still unknown, we have to rely on high-throughput, empirical exploration of the massive design space. Unfortunately, there is no feasible or affordable method for intervening at tens of thousands of loci in a genome beyond limited-use base editors or rudimentary knockout screens. So we down-select the possibilities we can test, leaving far too much to chance and the unexplored.
Consider a few common examples. Let’s say you scanned through research papers and databases and assembled a list of thousands of SNPs relevant to your project, and to understand which ones are causal for your trait or phenotype of interest, you’d like to recapitulate them in a clean background. Currently, that simply isn’t feasible. Even the best-funded labs might only be able to test a few hundred of them and at fairly high time and expense. In other cases, metabolic engineers would like to be able to carry out large-scale protein mutagenesis experiments by making many thousands of amino acid changes at numerous genomic locations relevant to a certain trait, for instance across entire biochemical pathways as well as genome-wide. But realistically, only a few loci can be targeted in these experiments with existing technologies. In yet another example, researchers seeking to edit regulatory regions across the entire genome can only target a limited number of locations. These types of changes require the ability to confer a wider variety of edit types since they are usually larger than the one to three nucleotides needed for SNP and codon changes.
Scaling up CRISPR means increasing the size and variety of edit types that can be made, as well as the overall number of edits and combinations of edits that can be performed at once. We need robust methods that yield high edit rates at scale. We also need significant improvements in the ability to perform multiplex combinatorial editing, which is essential for identifying relationships between sequence and function and accelerating gain of function. Making edits in combination over iterative cycles, an indispensable capability for plant and animal breeders and protein engineers alike, is out of reach for most labs today.
What’s Next
Thanks to CRISPR, biology is shifting from an observational science to an interventional science. Perturbing systems at the genomic level and studying the functional outcomes will help us better understand organisms, giving us critical information that we can use to improve health, increase crop yields and produce essential materials more efficiently and sustainably.
But we cannot get there with today’s CRISPR techniques. Together, we must encourage innovation around the fundamental methods used for CRISPR and, ideally, the development of automated approaches to make the process more robust. We need better design and tracking tools, paired with scalable, turnkey editing protocols, along with software, machine learning and other informatics tools to mine the data coming from large-scale experiments. Such capabilities will help us achieve the full potential of CRISPR-based genome engineering.
Richard Fox, PhD, is Executive Director of Data Science at Inscripta.
Tell Us What You Think!