Despite the buzz surrounding AI in the past decade, its implementation within scientific research organizations isn’t as pervasive as one might think.
One of the reasons for this is that research organizations often face a staffing dilemma when it comes to managing large-scale computational research and computational analysis. Historically, most research organizations have employed scientists who can write experiment code but may not possess deep expertise in engineering.
Machine Learning platforms, on the other hand, cater to technical users who are well-versed in command-line interface, git, docker, APIs, and version control. These skills are critical for conducting large-scale machine learning experiments, managing extensive datasets, collaborating with non-technical domain experts, and ultimately, forming a “chain of trust” for transparent, repeatable science that can be replicated from start to finish, beginning with project inception and going all the way through to final decision-makers and regulatory bodies. There is currently a scarcity of skilled machine learning engineers, dedicated DevOps teams focusing on creating and maintaining these systems, and personnel with a strong foundation in formal computer science.
And therein lies the paradox for research organizations: they need to handle increasingly complex, multi-modal datasets that standard pre-trained neural networks cannot process, but their researchers are not necessarily equipped to manage the very complex technical aspects associated with the de novo training and deployment of machine learning models on such datasets. Meanwhile, the individuals who do have this training (engineers, developers, administrators) aren’t necessarily familiar with scientific research topics and processes.
Traditionally, this has required research organizations to deploy teams of research scientists and data scientists to work in parallel. Although this model may have some short-term advantages, most organizations find that it ultimately leads to an inefficient allocation of time and resources.
This often presents a dilemma for research organizations, prohibiting them from taking full advantage of a data model or a technical platform’s functionality, or hindering them from even attempting to adopt AI.
A shared commitment to scientific discovery and open collaboration
The Allen Institute is a Seattle-based independent nonprofit bioscience and medical research institute founded by Microsoft co-founder and philanthropist Paul G. Allen. Composed of five scientific units and more than 800 employees, it conducts large-scale research through foundational science to fuel the discovery and acceleration of new treatments and cures for diseases such as Alzheimer’s disease, heart disease, cancer, addiction, and more. In keeping with its commitment to open science, one of The Allen Institute’s unique core values is to make all data and resources publicly available for external researchers and institutions to access and use.
As an associate investigator for the Allen Institute for Neural Dynamics (AIND), Dr. Jerome Lecoq serves as a principal contributor to OpenScope, a project that aims to share complex neuronal recording pipelines with the entire neuroscience community. His research applies large-scale deep learning, dealing with millions of data points that require extensive processing pipelines to handle and run analyses on complex and rich neuronal datasets. Because the OpenScope project is a highly collaborative and distributed effort, Dr. Lecoq’s team wants to share as much as possible about its data and processes with the broader neuroscience community.
Many ongoing projects at the Allen Institute, including OpenScope, use custom pipeline management code running on an on-premises High-Performance Computing (HPC) cluster. This workflow system was not designed to be exported to outside parties, a critical need of the OpenScope project. As a result, when it came to sharing Dr. Lecoq’s computational process with the broader neuroscience community — a key value of the Allen Institute — he was previously able to share only a small portion of his process, as opposed to the whole end-to-end workflow, because his pipelines were designed to run on the Allen Institute’s specific compute infrastructure.
In addition, Dr. Lecoq found that many research laboratories were eager to adopt processing pipelines validated in dedicated institutions like the Allen Institute, rather than having to replicate all processes from scratch in their laboratories. “So far, we have mostly not been able to leverage the opportunities presented by the cloud,” said Dr. Lecoq. “The neuroscience community is building over and over the same pipelines across hundreds of laboratories. Each one of these pipelines requires dedicated engineers, or more often students who must learn the entire code stack. It very likely slows our scientific progress.”
Helping research organizations to scale effectively
Dr. Lecoq used Code Ocean, a reproducible computational science platform, to address these challenges. He reports two benefits so far:
- Code Ocean’s “self-service” capabilities. Code Ocean has enabled Dr. Lecoq and other scientists to independently design and build pipelines, allowing AIND’s engineers to shift their focus from routine support tasks to more meaningful projects.
- Increased interoperability within the broader neuroscience community. Using Code Ocean, Dr. Lecoq’s team can share the full pipeline instead of just a portion.
Using Code Ocean has resulted in efficiency gains for The Allen Institute, as well as a reallocation of time and resources to higher-leverage activities:
- The speed at which a pipeline can be built has decreased from 12 to 3 weeks, a 4x increase in workflow efficiency.
- The Allen Institute has been able to reallocate the time of five engineers to more impactful projects.
- With fully interoperable Code Ocean Pipelines, the time and effort required to share a pipeline has been reduced to just one click.
Since implementing Code Ocean, the Allen Institute has seen a dramatic increase in interoperability and productivity. With more than 100 researchers actively using the platform, the organization has achieved a 400% increase in workflow efficiency, enabling the research teams to accelerate the pace of discoveries. “Code Ocean’s self-service capabilities make it easy for our scientists to do their work reproducibly,” said Dr. David Feng, director of Scientific Computing, Allen Institute for Neural Dynamics. “New users to the platform can get far with just a little support, giving our engineers time to focus on domain-specific challenges.”
Code Ocean has developed a wide range of dedicated features to assist large organizations such as The Allen Institute to operate effectively at scale. These features include fine-grained custom metadata management and search, data organization in Collections, automated data provenance for trusted science and reproducibility, and integration with external data warehouse providers and leading machine learning platforms. One noteworthy statistic is the number of times scientists committed code to git (2,370 times) and the percentage of users committing to git (61%). These figures surpass what’s typically observed within the broader scientific community (Ram, 2013). This achievement has been made possible by Code Ocean’s dedicated UI features, which bypass the need for command-line operations requiring expertise in git.
These statistics show that the Allen Institute not only reaps the benefits of implementing these foundational technologies at a broad scale but also reinforces industry-standard best practices. Among other benefits, Code Ocean has empowered the Allen Institute to use machine learning on a broad scale, for the first time handling petabyte-scale data, with a growing user base of 282 users running more than 15,000 computations, totaling over 10,000 hours.
“Our partnership with Allen Institute has helped us to refine our ability to accommodate the specialized needs of large organizations,” said Dr. Daniel Koster, vice president of Product at Code Ocean. “We have adapted our services to meet requirements such as handling petabyte-scale data, deploying FAIR-based analysis for reliable, scalable science, ensuring the reproducibility of scientific outcomes from experiment initiation to final presentation, complying with necessary regulatory standards, and providing a single pane of glass that integrates the multitude of tools utilized internally.”
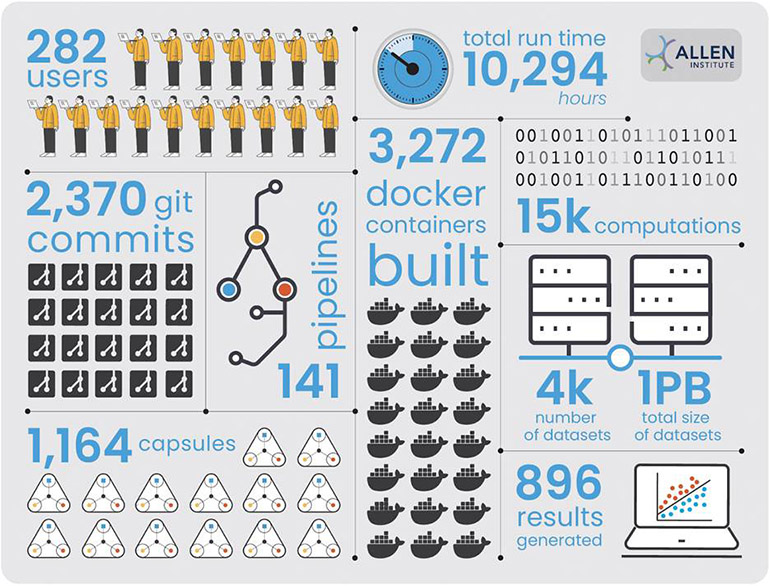
Less than a year into their partnership, the Allen Institute has already generated a data corpus on Code Ocean of over a petabyte, with a growing user base of 282 running over 15,000 computations totaling over 10,000 hours.
Code Ocean accelerates life sciences, pharmaceutical, and biotech by providing one trusted place for researchers to share research data and computational assets, collaborate more quickly, and communicate more effectively. The first and only integrated library and workbench that preserves a record of all coding, data, and software used in computational research, Code Ocean’s digital platform guarantees reproducibility by maintaining complete visibility of the research lineage.
One major thought, normal commercialization of a “lab built” product takes 10 years before the market is stable enough to really make good use of it, reliably…..laws built to contain it another 20 years….
Don’t you think it’s time that you addressed this up front? Isn’t it a little childish to think that the boom, bust, crash and burn cycle will somehow miss AI/ML?
Please note: I was talking about weighted paths/ML, Neural Net Training, Automated whatever/human replacement at a think-tank in the mid 1980’s….in McLean VA. I also worked R&D in St. Louis MO at the same place my father and his friends hammered out the basics of silicon production for chip manufacturing, lasers, and some other stuff…..
I also experienced the internet boom/bust/maturation cycle.
Perhaps you want to actually try to learn about how things mature in the market place before you launch a big boomerang of “what were you thinking?”
pick some niche markets that don’t destroy everyone’s future(s)….
How many word processor apps were around in the early days of computing, database applications? Sybase became Microsoft’s Database application package……you want to be swallowed by whoever has the most money to buy your stuff and rebrand it?
Control your own future…..plan, think, cooperate.